As emergency radiology section chief at a Level 1 trauma center, Jeffrey A. Hashim, MD, knows what it’s like to face a backlog of cases. Particularly when working overnight, Hashim says that cases can often pile up. In the past, with no way of knowing which cases were most critical, Hashim and his colleagues at Lahey Hospital & Medical Center in Burlington, Mass., would read through the worklist from top to bottom, quickly opening and scanning each case for urgent findings and hoping they didn’t miss anything in the process. It was all “a little anxiety provoking,” Hashim admits.
But now, Hashim and his team have help: A suite of artificial intelligence (AI) algorithms combined with workflow orchestration software is triaging cases and moving those with potentially critical findings — including pulmonary embolisms, intracranial hemorrhages, and cervical fractures — to the top of the worklist, alleviating some of the pressure on the radiologists. “I’ve definitely seen a decrease in my level of anxiety, because we know that these algorithms are operating in the background and will detect most of the things that we need to pay attention to right away,” Hashim says. “Minutes often matter in these situations. These tools give us peace of mind and are potentially helping us save lives.”
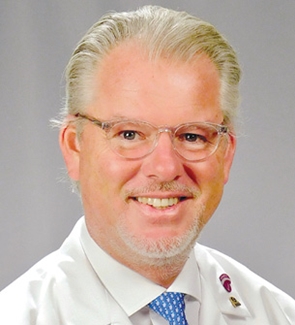
Christoph Wald, MD, MBA, PhD, FACR, chair of Lahey’s radiology department and chair of the ACR Commission on Informatics, led AI implementation within the practice.
|
Findings detection for improved workflow support is what most radiologists can expect as more practices begin integrating AI into their systems, says Christoph Wald, MD, MBA, PhD, FACR, chair of Lahey’s radiology department and chair of the ACR Commission on Informatics. “AI-generated results are increasingly influencing how radiologists prioritize their work,” he says. “This could be particularly relevant in scenarios where there is a mismatch between the number of studies to be read and the size of the workforce, for instance during night or weekend shifts when a large number of studies are directed to a smaller number of radiologists than during the regular business hours. The same might apply in certain teleradiology settings in which cases from many sites may be aggregated and presented to designated interpreting radiologists in consolidated reading lists.”
Recognizing an Opportunity
Radiologists at Lahey began integrating AI algorithms into their workflow in 2018. At the request of referring physicians, the first algorithm they implemented was one that helps detect stroke and shares the diagnosis with providers. “The stroke team asked us to deploy that tool as part of our comprehensive stroke center efforts,” says Wald, who is also a professor of radiology at Tufts University Medical School. “The solution had been used in most of the big stroke trials and was emerging as part of the standard of care in stroke.”
While the benefits of the stroke detection algorithm were proven, AI’s impact on radiology in general was unclear at the time, and the FDA had approved only a few algorithms for clinical use. Still, Wald was eager for his colleagues to experiment with the technology. “With the velocity of AI development and the promise it holds for assisting and complementing radiologists in their practice, I felt that we needed to expose our department to clinical AI implementation,” Wald says. “Our approach was mostly designed as a learning journey so that we could become familiar with the vendor contracts and understand firsthand how the technology works in the clinical setting.”
Wald and his team were particularly interested in implementing case prioritization tools, like those that Hashim and his colleagues use to triage cases that require immediate attention for potentially life-threatening conditions. “We felt that if there was any benefit to be had from AI while we were still figuring out how well it actually worked, it was in case prioritization,” Wald explains. “It seemed safe because our radiologists were never too far behind on the worklist anyway. We felt that we could pick up that benefit while we were learning about AI’s detection accuracy.”
Given AI’s potential advantages, Lahey’s radiologists were excited about the technology, but they were unsure how it would impact their work. “I’m sure a few were a little ambivalent at first and wondered, ‘How good is it? Will it replace radiologists?’” acknowledges Mara M. Kunst, MD, neuroradiology section chief at Lahey Hospital and Medical Center. “But the devil is always in the details, and the more we read about it and the more we experienced it, the more we found that AI is a tool that can assist us but not replace us. AI is designed to answer very specific questions. As radiologists, we must read the whole study because we’re the experts, and while the AI is good, it’s not 100% accurate.”
Contracting with a Vendor
Without any data scientists on staff to develop or deploy AI tools, Lahey’s radiologists decided to work with a vendor that Wald met through an ACR® event. Wald liked two things in particular about the vendor: It had one AI algorithm that was already FDA approved for detecting intracranial hemorrhage and several other algorithms in development, and it was willing to collaborate with the department’s worklist vendor to synchronize the algorithms and worklist for case prioritization. “I talked to a few companies and this one seemed easy to work with and agile,” Wald recalls. “Plus, they had a suite of tools under development that focused on critical findings, which was a pretty good match for what we do.”
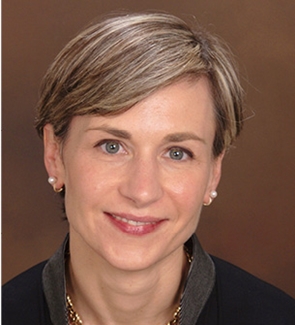
Mara M. Kunst, MD, neuroradiology section chief at Lahey, says that AI is a tool that can assist but not replace radiologists.
|
With a vendor in mind, Wald asked the radiology department’s information technology (IT) team to conduct a review to confirm that the company adhered to best practices, including using both a U.S.-based cloud data center for secure image processing and sophisticated data encryption technology to protect patient information. He also connected the vendor with the neuroradiology team, which gathered a few hundred cases to help evaluate the intracranial hemorrhage algorithm’s detection accuracy. “We were a testing site, so we were interested in seeing how well the algorithm performed,” says Kunst, who led the effort. “The results were in line with what the vendor advertised — with sensitivity and specificity between 90-95%.”
Once the IT team finished its review and the neuroradiology team completed its testing, Wald and other radiology leaders negotiated a price and signed a contract with the vendor. Over the ensuing two years, they worked with the vendor to integrate six algorithms into the department’s workflow as the algorithms received FDA approval.
Integrating the Algorithms
At the start of the integration process, the AI and worklist vendors collaborated to sync their tools for case prioritization. “We wanted to appropriately move those cases that the AI algorithms flagged with positive findings to the top of the worklist so that we could prioritize and read them more quickly,” Hashim explains. “We also needed to see the AI findings clearly, so the vendors created a red (positive) and green (negative) badging system that is visible next to the patient name within the worklist. That way, the radiologist would know whether the AI had detected either a positive or negative finding in the study in one glimpse at the worklist.”
The radiologists also discovered during the post-integration phase that they needed to know when an algorithm was still processing a study; otherwise, they would sometimes sign off on a case before the AI had analyzed it — which resulted in a potential missed opportunity to leverage the technology, Wald says. To that end, the vendor added an additional gray badge that indicates when the AI is processing a case. “As a radiologist, you don’t want to sign off on a report and then have the AI come back saying that it found something that you might have missed,” Hashim says. “You want to have an opportunity to consider the AI output and include any findings in your report as appropriate.”
For this reason, the practice introduced another safeguard: When an AI result arrives after the corresponding imaging study report is “final,” an obvious alert pops up on the screen of the radiologist of record. This message lets the radiologist know that an AI result is now available on a study they have already signed off on. From there, the user interface offers the radiologist a one-click option to return to the study, facilitating additional review of the images with knowledge of and the ability to correlate with the AI result.
In addition to syncing the algorithms with the worklist, the team wanted to ensure that the AI never posted its representative findings to the hospital’s picture archiving and communications system (PACS) to avoid other providers seeing the outputs before the radiologists. “The moment a result posts to the PACS, it is visible to the entire healthcare enterprise,” Wald explains. “You have no idea who sees it, when they see it, what conclusions they draw from it, and what clinical actions they take. We wanted to ensure that the radiologists could review the outputs and decide whether to incorporate them into their reports. At this point, radiologists use only the vendor’s custom viewer to review AI results and keep all of the AI findings within radiology.”
Working with AI
Even with several algorithms now integrated into their workflow, the radiologists’ work remains relatively unchanged. They still read every case. The only difference is that they read the cases that the algorithms flag for positive findings sooner than they might have otherwise, and they consider the AI outputs in the process. “We’ve established a nice workflow, using the AI as a second reader and checking to see if it’s caught anything before we sign off on our reports,” Kunst says. “It’s a nice tool to have in your back pocket.”
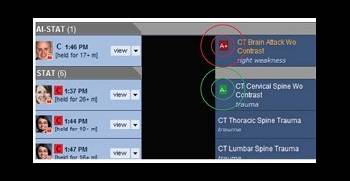
The AI and workflow vendors created a red (positive) and green (negative) badging system that clearly displays the algorithms findings.
|
In some cases, the algorithms have detected findings that the radiologists have missed. For instance, before the vendor instituted the processing badge, Wald recalls signing off on a case that the algorithm later flagged for pulmonary embolism (PE). When he reviewed the images again, Wald realized that the AI had noted a small PE that he had missed.
“In an instance like that, you call the referring clinician and let them know that you’ve changed your mind about the results,” Wald explains. “It’s just like when a colleague sees your case the next day in the peer learning system or conference, and they see something that you did not see. It’s not uncommon to fine tune a report shortly after it was made based on additional clinical information or colleague feedback. Usually this requires additional communication and documentation but doesn’t have a significant adverse impact on patient care. On the contrary, radiologists should never leave an opportunity on the table to improve their report and the care it informs.”
While the AI has occasionally bested the radiologists, it has more frequently missed the mark by reporting false-positive findings. The radiologists track how well the algorithms perform using a feedback loop that the vendor incorporated into the system. If the radiologist agrees with the AI, they note the finding in their report and click a concordance button within the system. If they disagree, they omit the finding from their report and click a discordance button within the system.
“This feedback gives the vendor access to a radiologist-generated ground truth for how their algorithms are actually performing in the real world,” Wald says. “The more the vendor can learn about how their algorithms are performing, from both a usability and functionality standpoint, the more opportunity exists for them to develop tools that help radiologists add additional value to the care team and improve future versions of the algorithms.”
Understanding the Benefits
The vendor isn’t the only one learning when the radiologists use the algorithms. The radiologists may benefit as well. For instance, if the algorithms flag findings in a particular area of an imaging study that a radiologist has overlooked in their initial review, the radiologist knows to double-check that area before signing off on their reports, Kunst explains. “It can be mutually beneficial: You give the AI feedback and the vendor learns, and with careful retrospective analysis, it may help radiologists learn which areas require closer attention,” she explains. “It really becomes a partnership and can add to our peer learning efforts.”
This partnership ultimately leads to improved patient care because the radiologists can do their jobs better and more efficiently, as has been the case in the emergency room. “Our section was struggling with case prioritization, especially when only one of us is working at night and two or three traumas hit the deck at the same time,” Hashim explains. “With our commitment to excellent patient care, we really needed to solve this problem. These algorithms help us identify acute findings more rapidly so that we can communicate them to the clinical team, which can take interventions early and aggressively.”
Although the algorithms work well for workflow prioritization and show promise in other areas, the tools won’t displace radiologists anytime soon, Wald says. “Most of the time, radiologists are perfectly capable of making all of the key diagnoses, but occasionally they benefit from having the AI,” he says. “Radiologists report that they like the ‘second look’ nature of the technology. But like any other tool, AI has limitations. It’s a good tool for addressing specific tasks, but it certainly doesn’t work well enough to replace the radiologist.”
Adding More Tools
With the successful integration of these algorithms, Lahey’s radiologists are considering adding more AI tools to their workflow. Wald is especially interested in algorithms that can do things that are difficult for radiologists to execute alone but that are meaningful to referring physicians and patient care, such as lung emphysema or liver fat quantification. “We’re focused on algorithms that can perform quantitative analysis and other tasks that we, as radiologists, cannot easily do,” he explains. “That’s a big value add that matches the current and future demand in our practice. AI is expensive to integrate so we want to be sure that we adopt algorithms that help us improve care, complement our professional work, and enhance our work product.”
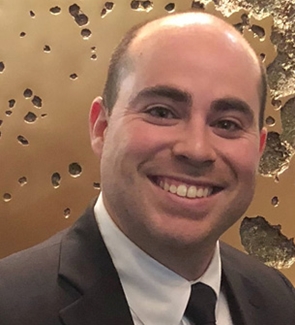
Jeffrey A. Hashim, MD, emergency radiology section chief at Lahey, says the AI algorithms help his team prioritize cases with potentially critical findings. |
Wald recommends that other practices also take a thoughtful approach when adopting AI. To help, he says, the ACR Data Science Institute has created
AI Central™, a catalog of FDA-approved algorithms and supporting materials. “The FDA has already cleared more than 110 algorithms, so groups should definitely take time to compare them and figure out which ones will best help them achieve their goals,” Wald contends. “AI Central includes the FDA clearance summaries, information about the AI marketplaces in which a specific algorithm can be found, performance indicators from the submission documentation, and vendor contact information to help radiologists make informed decisions about which AI algorithms they might want to consider.”
While Wald and his team advocate that radiologists take a measured approach when adopting AI into their workflows, they encourage all radiologists to familiarize themselves with the tools and learn how to leverage them for better patient care. “It’s a good idea to get comfortable with the technology because it’s going to be around in the future,” Hashim says. “When integrated properly, these tools can add meaningful value to our profession. We must embrace this technology to ensure we’re doing all we can to help the patients we serve.”